Your AI Might Be Misleading You: Understanding the Dual Nature of LLM Outputs
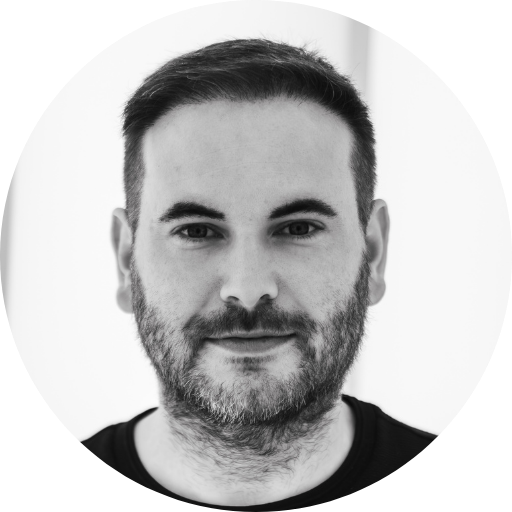
Dimitri Allaert
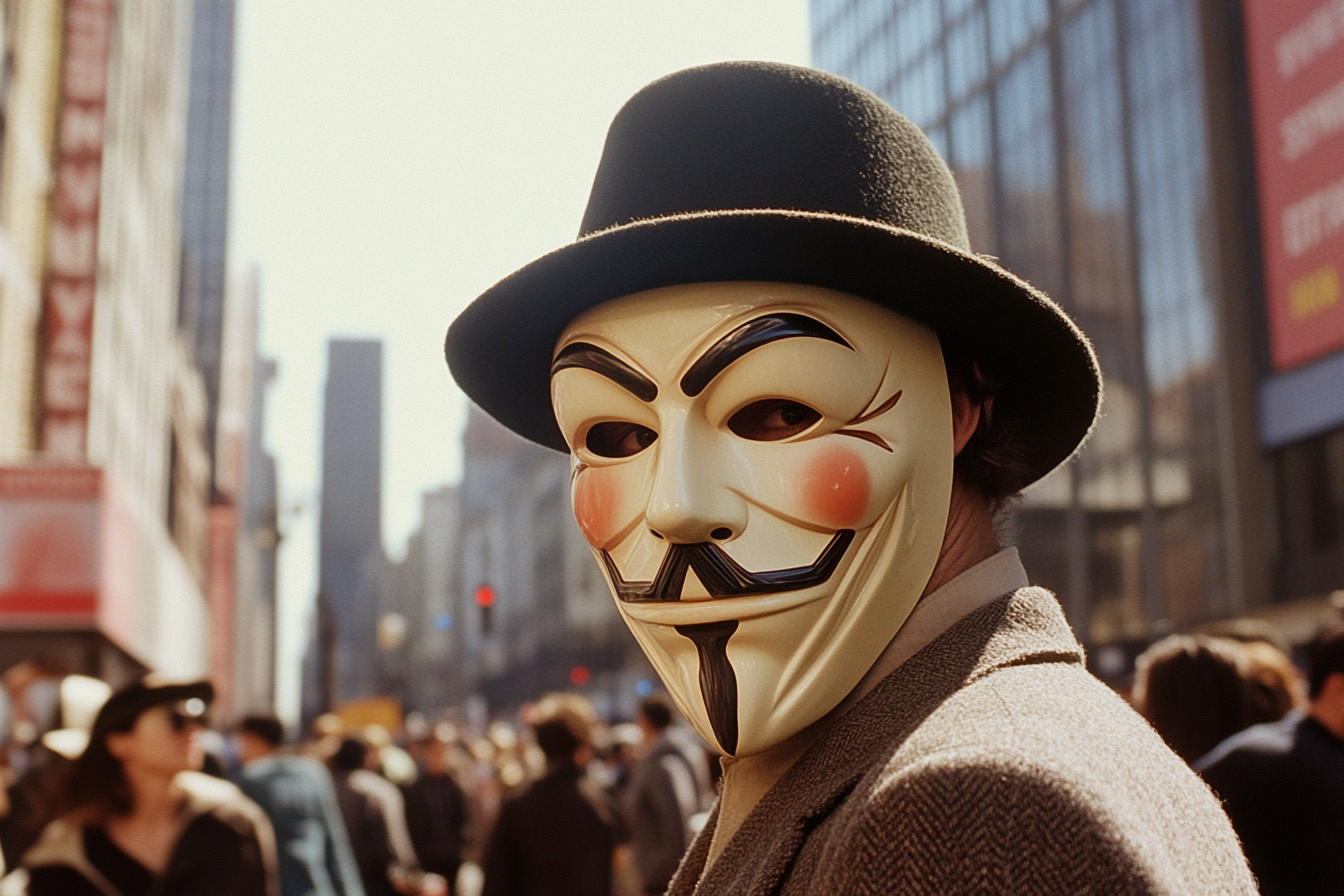
In the rapidly advancing world of artificial intelligence, it's easy to trust the outputs of sophisticated Large Language Models (LLMs) like GPT-4. However, even when these models produce responses that are factually correct, they can still mislead. This paradox arises because LLMs, despite their impressive capabilities, often generate information that lacks crucial context, presents incomplete data, or overgeneralizes, leading to conclusions that might not be fully accurate.
For example, an AI might correctly state that "Electric cars produce zero emissions," but fail to mention the emissions from manufacturing and electricity production, presenting a skewed view. Similarly, while running can improve cardiovascular health, it's not universally safe, particularly for those with specific medical conditions. Such nuances, when omitted, turn a technically true statement into a potentially misleading one.
Businesses relying on AI must be aware of these pitfalls and take proactive steps to ensure that the outputs of these models are both accurate and contextually relevant. One effective approach is using Retrieval-Augmented Generation (RAG) systems. These systems enhance AI responses by pulling in verified, relevant information from a curated database, ensuring that what the AI says is not only true but also complete and reliable.
At Vectrix, we are committed to advancing RAG systems to minimize the risks of AI-generated misinformation. By focusing on accuracy, transparency, and continuous improvement, we strive to provide AI solutions that deliver trustworthy and meaningful insights.
What You Will Learn When Reading the Full Blog Post
In our full blog post on Medium, we dive deeper into the nuances of how LLMs can produce outputs that are both true and misleading, and why this is a critical issue for anyone relying on AI. We explore real-world examples and discuss advanced strategies, such as Retrieval-Augmented Generation (RAG), to mitigate these risks. While the content is slightly more technical, it remains accessible, offering a comprehensive understanding of the dual nature of AI-generated content and practical solutions to enhance the reliability of these powerful tools.